Dr. Bhusan Chettri a Ph.D graduate from Queen Mary University of London (QMUL) giving an overview of Voice Biometrics, Spoken phrase dependent and phrase independent voice biometrics & Countermeasures or anti-spoofing solutions to prevent fraudulent access
London, United Kingdom, 28th Jul 2022, King NewsWire, Each person has a unique voice with distinctive features that can be used to automatically verify a person’s identity. Voice is considered as one of the most convenient form of biometric authentication solution among other biometric identifiers such as face, fingerprints and iris. Voice biometrics use speaker verification technology and the main goal here is to recognize humans automatically using their voices. It comprises two tasks: speaker identification and speaker verification. Speaker identification involves finding the correct person from a given pool of known speakers or voices. A speaker identification usually comprises a set of N speakers that are already registered within the system and these N speakers can only have access to the system. Speaker verification on the other hand involves verifying whether a person is who he/she claims to be using their voice sample. These systems are further classified into two categories depending upon the level of user cooperation: text dependent and text independent systems. In text-dependent applications, the system has prior knowledge of the spoken text and therefore expects the same utterance during deployment phase. For example, a pass-phrase such as “My voice is my password” will be used both during speaker registration and deployment (when the system is running). On the contrary, in text-independent systems, there is no prior knowledge about the lexical contents, and therefore they are more complex than text- dependent ones. Figure 1 and 2 summarises the speaker enrollment (registration) and deployment (testing) phases of voice biometrics.
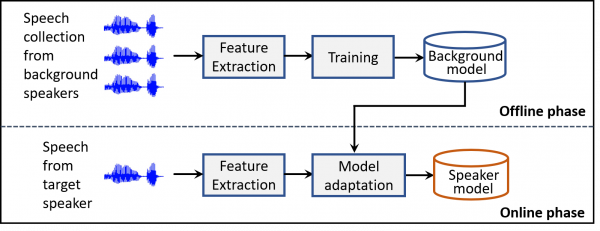
Figure 1. Speaker enrollment phase. The goal here is to build speaker specific models by adapting a background model which is trained on a large speech database.

Figure 2. Speaker verification phase. For a given speech utterance the system obtains a verification score and makes a decision whether to accept or reject the claimed identity.
“Even if voice biometrics is among the most convenient means of biometric authentication, their robustness and security in the face of spoofing attacks a.k.a presentation attacks is of growing concern, and is now well acknowledged by the speech community.. A spoofing attack involves illegitimate access to personal data of a targeted user. The vulnerability of voice biometrics against spoofing attacks is an important problem to solve because it poses a serious threat to the security of such systems. When successful, a spoofing attack can grant unauthorized access of private and sensitive data”, says Bhusan Chettri. Spoofing attack methods include Text-to-Speech, Voice Conversion techniques, impersonation and playing back speech recordings. High-stakes biometric applications, therefore, demand trustworthy fail-safe mechanisms (countermeasures) against such
attacks. A countermeasure (CM) in voice biometric context is a binary classifier that aims at discriminating bonafide (human speech) utterances from spoofing attacks (fake speech). To allow maximum re-usability across different applications, the ideal CM should generalise across environments, speakers, languages, channels, and attacks. In practice, this is not the case; CMs are prone to overfitting. This could be due to variations within the spoof class (e.g. speech synthesizers or attack conditions not present in the training set), within the bonafide class (e.g. due to content and speaker), or extrinsic nuisance factors (e.g. background noise).
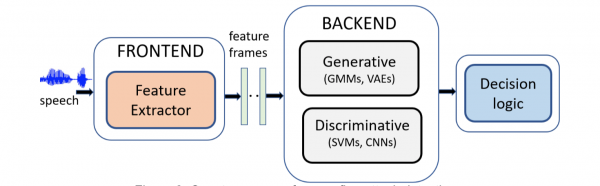
Figure 3: Countermeasure for spoofing attack detection
Like any traditional machine learning classifier, a spoofing countermeasure (Fig. 3) typically consists of a frontend module, a backend module and a decision logic (for the final classification based on a decision threshold). The key function of the frontend is to transform the raw acoustic waveform to a sequence of short-term feature vectors. These short-term feature vectors are then used to derive either intermediate recording-level features (such as i-vectors) or statistical models, such as Gaussian mixture models to be used for bonafide or spoof class modeling. In contrast to these approaches that require a certain level of handcrafting especially in the frontend, modern deep-learning based countermeasures are often trained using either raw-audio waveforms or an intermediate high-dimensional time-frequency representation — often the power spectrogram. In these approaches, the notions of frontend and backend are less clearly distinguished.
Media Contact
Organization: Bhusan Chettri
Contact Person: Bhusan Chettri
Email: Send Email
City: London
Country: United Kingdom
Website: https://bhusanchettri.com/
The post An overview of Voice Biometrics by Bhusan Chettri appeared first on King Newswire.
Information contained on this page is provided by an independent third-party content provider. Binary News Network and this site make no warranties or representations in connection therewith. If you are affiliated with this page and would like it removed please contact [email protected]